
Here the authors adopt a convolutional LSTM ( ConvLSTM) layer and a warping loss. The third mechanism is based on the temporal memory layer - to help connect internal features from different time steps in the long term. While the recurrent feedback connects the consecutive frames, filling in the large holes requires more long-term knowledge. The second mechanism includes explicit flow supervision at the finest scale between two consecutive frames with FlowNet2. To achieve this, flow sub-networks is used to estimate the flows between the feature maps at four spatial scales (1/8, 1/4, 1/2, and 1). This strategy helps the model borrow traceable features from neighbouring frames. Before being combined, source and reference feature points are aligned. The first mechanism is based on feeding in the generated frame from the previous step along with the frames in the current step - recurrent feedback. It should be should be consistent with spatio-temporal neighbour frames X(t+/-N) where N denotes a temporal radius the previously generated frame Yt−1 and all previous history. Authors set three requirements for the generation of the t-th frame. The narrative of the paper builds around ways of ensuring temporal consistency of the inpainted video.
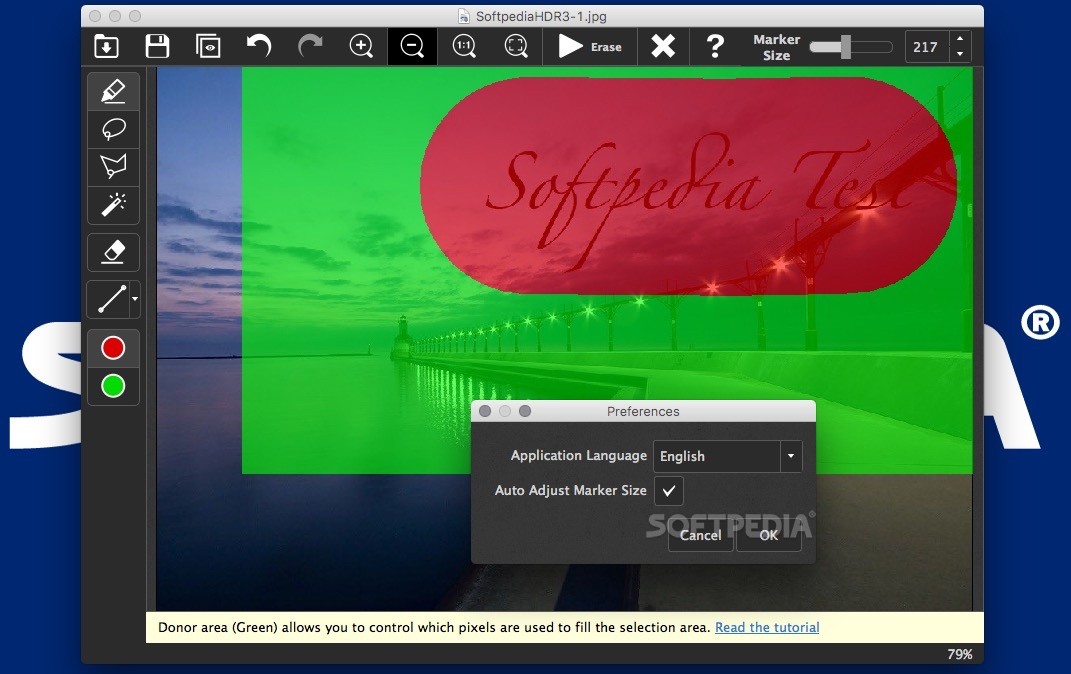
Since computational complexity of optimization-based methods for flow-estimation is high, many methods described below either find mechanisms to replace, simplify or approximate it. If we cannot borrow the content to recover the current frame from the nearby frames, the still-unknown regions can be synthesized with single image inpainting for a single frame and then propagated to the rest of the video.īelow I go over the copy and paste architectures in the chronological order. For a quick explanation of optical flow methods check out this article. That is why backward and forward flow often do not match and often both are computed and joint information is used to refine the estimates. when the displacement is computed from the first frame forward, or from the last in reverse order.įinding optical flow is challenging, as there are might be multiple plausible solutions. Optical flow can be forward and backward – i.e. If the flow is computed with little error we can trace the exact position of a specific pixel from the first till the last frame. Optical flow provides information about displacement of the background and objects across frames. This is typically done with optical flow. To copy the contents of the missing pixel from the nearby frame we first need to trace the position of that pixel. With the latter being not as popular due to memory and time constraints. Generally proposed solutions employ Convolutional Neural Networks (CNNs), Visual Transformers or 3D CNNs. At the same time, generative methods might not be as accurate in reconstruction, often producing blurry, averaged across possible options, solution. In this case generative models would be more suitable. Copy and paste methods work well in scenarios where pixels can be tracked through the video and would obviously fail when the information cannot be retrieved, for example a stationary video with a stationary region removed.
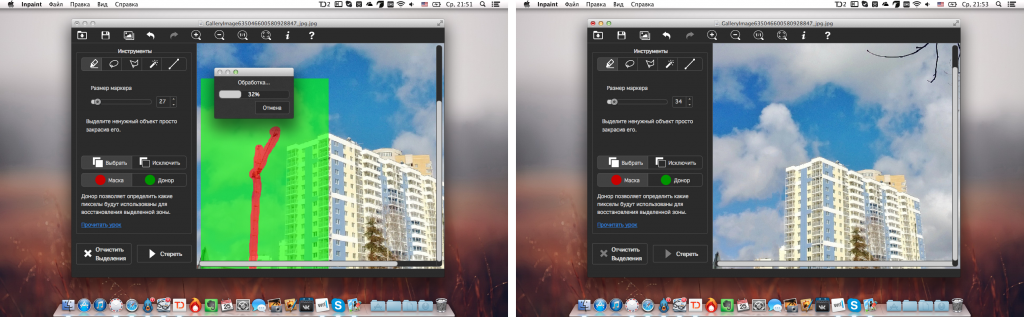
They can either be copy and paste – where the information for the missing pixel is searched for in the nearby frames and once found copied to the specified location, or generative, where some form of a generative model is used to hallucinate the pixel information in the region based on the content of the whole video.īoth methods have their pros and cons.
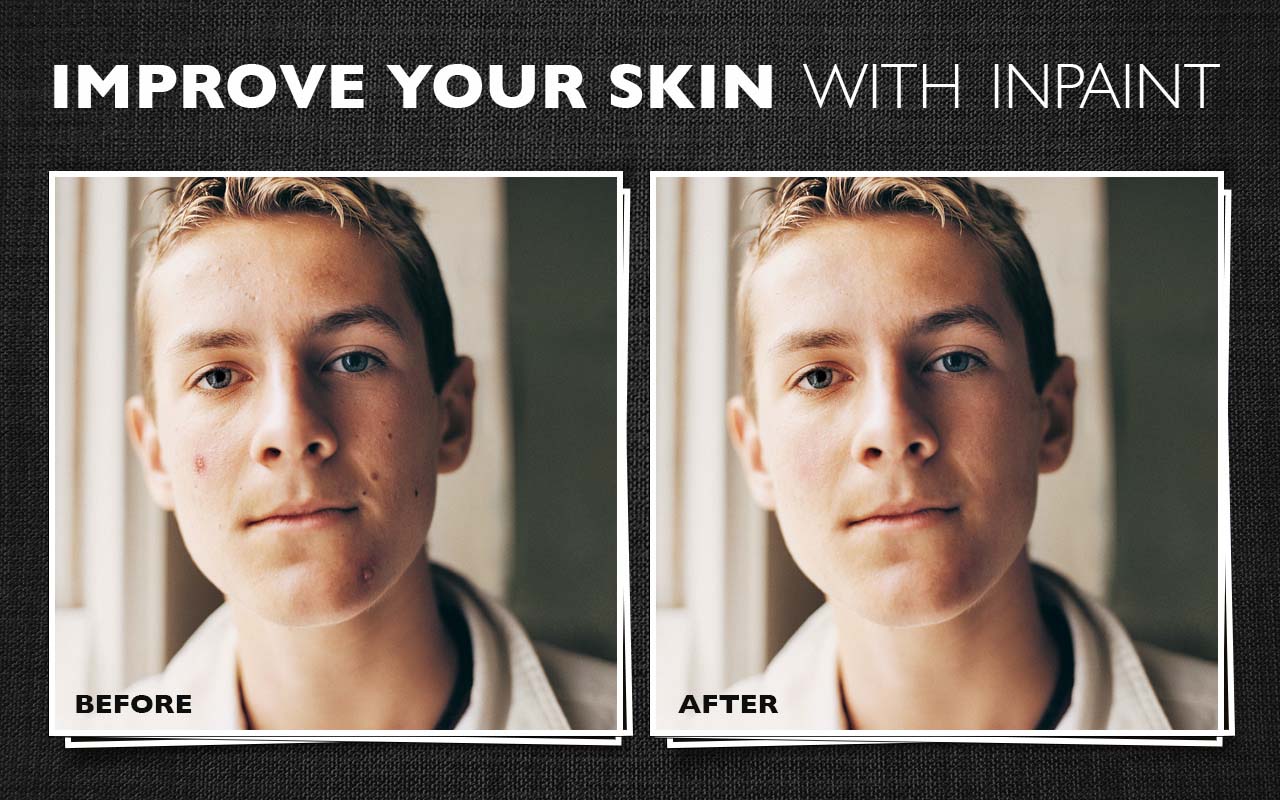
Generally available methods can be distinguished by the inpainting mechanism. While video inpainting is more challenging compared to image inpainting - due to the need to satisfy temporal-consistency between the frames, it inherently has more cues as valid pixels for missing regions in a frame may appear in other frames. And there are many variables that impact how difficult it is to achieve temporal consistency - complexity of the scene, changing camera position, change in the scene or movement of the selected area for inpainting (for example a moving object). Gradually changing over time video should not have flickering artifacts or sudden change in colors or shapes of objects in the inpainted region. Video inpainiting is conceptually similar to image inpainting, however, with a slight complication - the need to satisfy temporal consistency across the whole video. And Photoshop has replaced foreground (the selected area) with the background texture using “ Content-aware fill”.įor a video this process would be more laborious, as we would need to mark all frames where the object occurs. In masking this image I specified the region that I want to inpaint. Example of image inpainting with Adobe Photoshop.
